Part 1: Gender Bias In Futuristic Technology — AI In Pop Culture
The world runs on the assumption that numbers can’t be wrong; that data is objective and only works on merit. But what falls through the cracks is that systems don’t have a righteous mind of their own but rather develop a reason from the data it is fed. We tend to oversee that these codes reflect one’s own inherent biases, experiences and attitudes. As Caroline Perez, a feminist and author of Invisible Women says, that because of the way machine-learning works, when you feed it biased data, it gets better and better—at being biased.
Historically, the values of gender-caste-based minorities have been systematically kept aside from being even counted, which leads the prior data to be gender-biased or rather gender-invisible. As AI matures to further solo, smart performances, it essentially diminishes human dignity to a mere data point, indifferent to the inequities we are trying to conquer with AI in the first place.
Data scientists have pointed out broadly two ways in which AI perpetuates gender bias. The first one is caused due to Algorithmic and Design bias/flaw leading to unfair decisions made towards people of a certain gender, ethnicity, religion, geographic location etc. This can be understood as gender in technology in feminist frameworks. The other is the reproduction of gender stereotypes with new digital products projecting a gender of technology.
What causes datasets to be skewed?
Unnoticed algorithmic bias coupled with the massive scale of its impact can be life-altering. There have been several noted explanations to why the Artificial Intelligence systems are propagating gender bias. Known as Implicit bias in AI, it renders the whole decision-making system unfair at the very first step when the coder or the designer unconsciously designs machine learning models based on their own stereotypes.
Known as Implicit bias in AI, it renders the whole decision-making system unfair at the very first step when the coder or the designer unconsciously designs machine learning models based on their own stereotypes.For example – A woman is 47% more likely to be seriously injured and 17% more likely to die in a car accident compared to men. That is because most of the car seats are designed according to taller and heavier male bodies and the crash testing was, until recently, performed only using male dummies.
It influences the further stages of sampling and analysis as well. For example – A woman is 47% more likely to be seriously injured and 17% more likely to die in a car accident compared to men. That is because most of the car seats are designed according to taller and heavier male bodies and the crash testing was, until recently, performed only using male dummies. The car being designed by men for male bodies ignores the physical attributes of women’s bodies, even teenagers, disabled, obese and elderly for that matter.
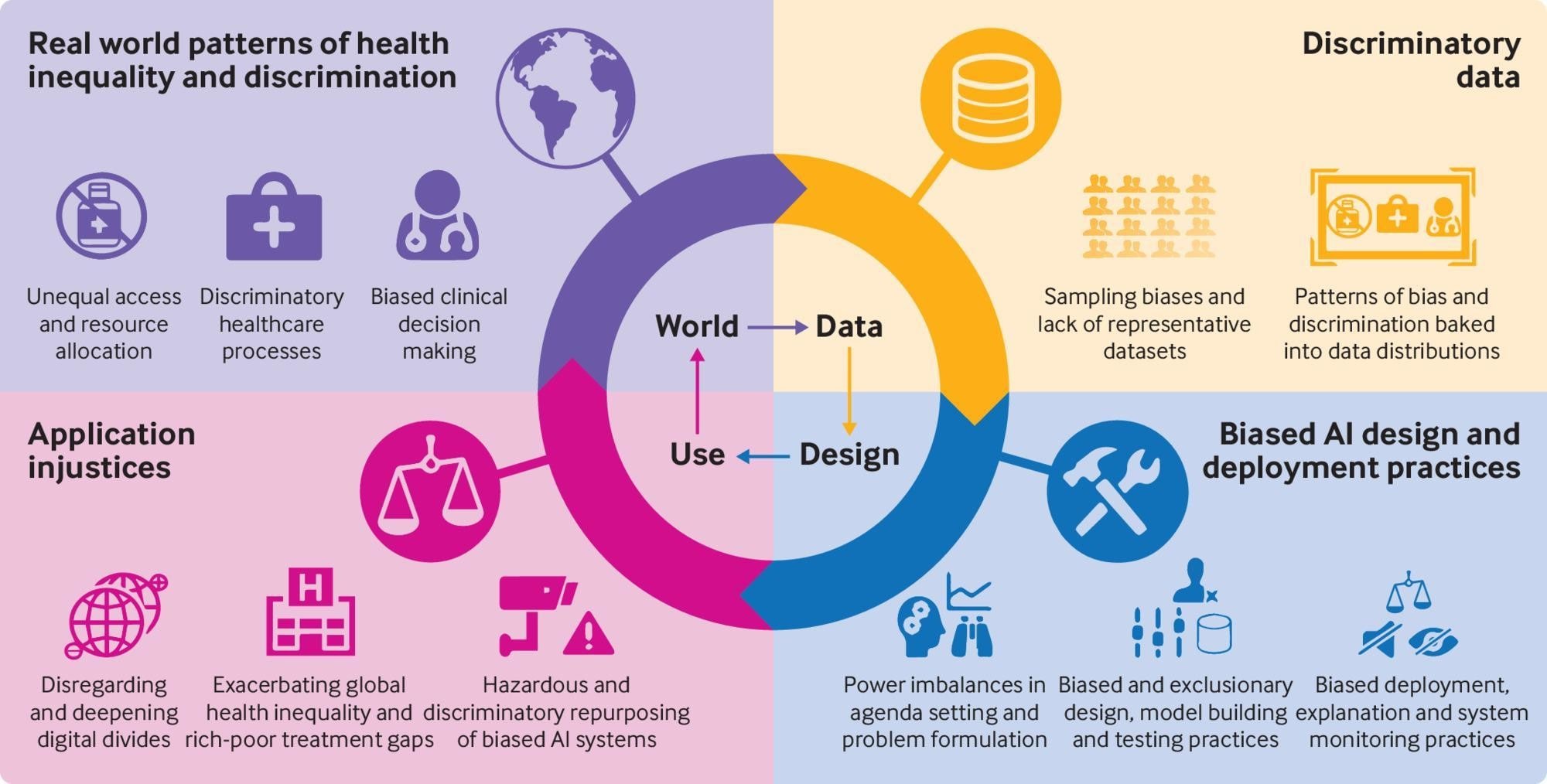
Another one of the more prominent explanations is the Coverage Bias in training datasets. Most often, the data fed to the algorithms are incomplete or rather do not reflect the population at large (or the population it is supposed to be making decisions for) in reflective proportions. A publicly criticized example of this – A 2015 drug trial to study the effect of alcohol on Flibanserin, popularly referred to as ‘Female Viagra’, had 23 male participants and only 2 female participants. The drug was intended to enhance libido among premenopausal women but the impact irrespective of alcohol consumption was reported none to low. The drug was approved by the FDA the same year nonetheless and is currently sold under the brand name of Addyi.
The AI systems, in consequence, likely mirror the digital divide among demographic groups. More often, the historical data used to train the algorithm is exclusionist from the past practices and hence could be ranking historically disadvantaged groups lower in line for opportunities. A famous example of this bias was reported in 2018, pointing out that the hiring practices of the e-commerce giant Amazon were biased towards women since the past ten years of recruitment data was dominated by men in technical roles. With extensive research, it was found that the algorithm down-ranked candidates who used the term “women’s chess team”, “women’s college” etc., in their CVs irrespective of the proof of qualification. Needless to say, when it was brought to light, the company had to disband the group which created the algorithm.
Another marked reason for troublesome algorithms can be Confirmation Bias – wherein a researcher tends to sample or interpret data that will approve his own pre-existing opinions, limiting them to explore more possibilities and questions. Other reasons are Sampling bias – where data wasn’t collected in a random manner from the entire target group, which would have ensured the most generalizable results. Participation bias occurs when the group’s heterogeneity in terms of the difference in the participation/drop-out rates of specific population categories, is not accounted for. Apart from the flaws mentioned above in the data, another notable discrimination in Big Data may occur through the ignored impact of ‘Outliers’. In decision-making situations, the outliers can be a minority or lesser-represented groups.
With the extensive commercialization of AI systems, it is imperative to note that a discriminatory algorithm that disapproves people on age, race, pin code, gender, sexual orientation etc., is hampering their human right to equality and eventually repeating violations of the past. A unilateral, toxic tech atmosphere presumes that its users are monoracial, able-bodied and heteronormative. More so, as we become more aware of the spectrum of gender and sexuality identities, these lesser-counted groups are very likely to be labelled as atypical and, in the process, disadvantaged by the system itself.
A unilateral, toxic tech atmosphere presumes that its users are monoracial, able-bodied and heteronormative. More so, as we become more aware of the spectrum of gender and sexuality identities, these lesser-counted groups are very likely to be labelled as atypical and, in the process, disadvantaged by the system itself.
Triangulation of Solutions
Artificial Intelligence is ready to impact Healthcare, Automotive and Financial services sectors with the maximum potential by 2030, which has utmost scope for product enhancement and positive disruption. And while the creators will have an obvious superiority with user preferences, with more customized solutions, they will also kick-out several slower competitors out of the market. Using Big Data for a positive future of the machine-human relationship is crucial as ever.
The governance of such a hegemonizing industry will be the trouble of the future. It brings to the worry several questions on digital human rights, privacy, right to non-discrimination, which this industry will likely soon pose unmanageably. And unless dealt with it uniquely, it dangers the progress made by several waves of the Feminist movements, Civil Rights movement, minority rights and LGBTQ+ rights movements globally in the mirage of a tech-inclusive society in the near future.
Seeing that the AI transcends disciplines and industries, reducing its bias will need to be a triangulation of methods. A reasonably basic solution to combat the problem is – gender segregated data. The bias stems from a culture of lackadaisical ‘unthinking’ about the need for women’s data because a default human almost always refers to a man. The ‘male default’ problem is responsible for several of the nearly fatal hazards for women in daily life. But ensuring that our data is intersectional and disaggregated is a challenge that requires us to solicit gender and racial diversity among creators at the very start of the game.
A crucial component of understanding AI and its repercussions is to be able to comprehend the workings of the AI system, which is producing decisions based on data. Several commercial algorithms using artificial neural networks and deep learning tools are dealing with Black Box AI problem. This is where even the programmer can’t explain how a particular result was brought on by the machine and is unnoticeable until something grossly incorrect or unexpected is observed.
Artificial neural networks are said to be similar to human neural networks of the brain and judgements are made by inherent identification of patterns and examples of the past, which continues to remain unexplained. In the wake of uncertainty and ethical concerns, simpler, more interpretable forms of AI should be preferred. It may not produce as advanced outcomes as higher models of AI but can be explained for its ethicality.
Also read: Geetha Manjunath, The Scientist Who Built AI-Driven Breast Cancer Detection
Tricia Wang, a tech ethnographer, proposed another resolution of the problem that dwells on the need to incorporate thick data along with big data. With the exponential growth of Big Data, encasing all its capabilities, an issue which Wang describes as the ‘Quantification Bias’ seems to have surfaced. This is the inherent bias of valuing the measurable against the immeasurable and qualitative. Data limits our worldview through a rather unilateral, positivist approach. She has proved that leveraging the best of human intelligence with qualitative and behavioural insights is key to obtaining a more holistic picture.
Tricia Wang, a tech ethnographer, proposed another resolution of the problem that dwells on the need to incorporate thick data along with big data. With the exponential growth of Big Data, encasing all its capabilities, an issue which Wang describes as the ‘Quantification Bias’ seems to have surfaced. This is the inherent bias of valuing the measurable against the immeasurable and qualitative. Data limits our worldview through a rather unilateral, positivist approach. She has proved that leveraging the best of human intelligence with qualitative and behavioural insights is key to obtaining a more holistic picture.
Cathy O’neil further articulated in her popular TED Talk that, “You need two things to design an algorithm – past data and a definition of success”. Hence, according to her, a regular check on both the previous data’s integrity to prevent algorithmic bias, as well as, auditing the definition of success for fairness can significantly reduce the amplification of existing bias. This brings into enquiry who is answering the questions? What questions are they asking? Who is designing these new systems? Who controls what data is used for training the products? Are the makers following the standards of AI Ethics and Gender equality? Do they care?
Automated systems need to be designed and trained to respect and recognize the diversity of our world rather than endangering it. Globally, STEM and digital skills education ecosystems are masculinized, leading to severe underrepresentation of minority groups in technology products. And while male-dominated data may not be an apparent problem to gender data bias, what comes out even more robust through this gender-based inquiry process, is that a feminist perspective is crucial to inclusivity. Several hundred years old patriarchal systems cannot be allowed to manifest themselves in latent forms through masculinely-devised tech products. There is an urgent requirement for more women on tables to create and manage futuristic, data-driven innovations. Therefore, the representation is not merely a technological issue or a moral need but rather, a personal and political imperative.
Mona is a researcher and feminist working at the intersections of gender, social policy and public health and writes contemporary stories using data. She holds a post-graduate degree in Women’s Studies from Tata Institute of Social Sciences and currently works with the Sustainable Development Programme at Observer Research Foundation. You can find her on LinkedIn and Twitter.